I’m overjoyed to announce the release of my latest book “The Economics of Data Analytics, and Digital Transformation.” The book takes many of the concepts discussed in this blog to the next level of pragmatic, actionable detail. Thanks for your support!
Organizations have a tragic mismatch between their investments in managing and governing data versus their investments in monetizing data. Organizations have invested vast amounts of money in enterprise, social and mobile systems to acquire, manage and govern customer and operational data, but lack a cohesive business strategy for monetizing said data (see Figure 1).
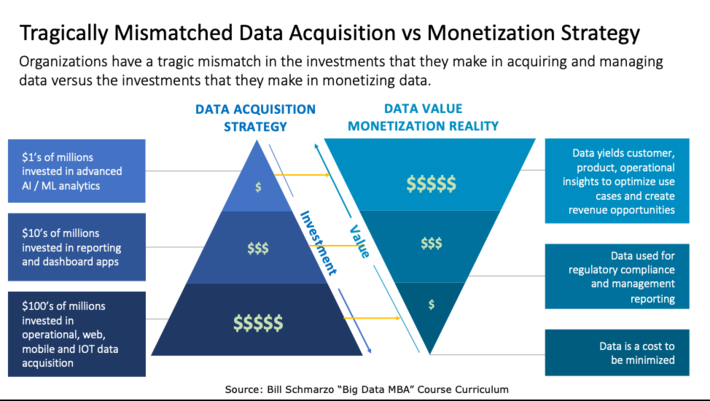
Figure 1: Tragically Mismatched Data Acquisition vs Data Monetization Strategy
This mismatch exists because most organizations lack a critical role – the Chief Data Monetization Officer (CDMO) – who is responsible and accountable for guiding the organization’s data and analytics developments in order to derive and drive new sources of customer, product, and operational value… with a business outcomes-centric approach.
If we truly believe that data is the new oil – that data will be the catalyst for economic growth in the 21st century – then we need to spend less time and investments trying to manage and govern data, and dramatically increase the time and investments to monetize data.
Redefining the Data Governance / Data Management Practice
I recently had the opportunity to keynote at Stanley Black & Decker’s Artificial Intelligence, Data & Analytics Summit (thanks Bala for the invitation and collaboration!). In preparation for the summit, Bala and I had some great conversations about the responsibilities of a data governance / data management practice in supporting the modern business. Today’s data governance and data management practices must be redefined to support the organization’s business needs and ultimately underpin the organization’s data monetization strategy. A Data Monetization practice must:
- Evangelize a compelling vision regarding the economic potential of data and analytic assets to power an organization’s digital transformation.
- Educate senior executives, business stakeholders and strategic customers on how to “Think Like a Data Scientist” in identifying where and how data and analytics can deliver material business value.
- Apply Design Thinking and Value Engineering concepts in collaborating with business stakeholders to identify, validate, value and prioritize the organization’s high-value use cases that will drive the organization’s data and analytics development roadmap.
- Champion a Data Science team to “engineer” reusable, continuously-learning and adapting analytic assets that support the organization’s high priority use cases.
- Develop an analytics culture that synergizes the AI / ML model-to-human collaboration that empowers teams at the point of customer engagement and operational execution.
The CDMO needs to own the Data Monetization practice; to understand the organization’s desired business and operational outcomes, and then create a pragmatic, actionable, iterative plan that delivers the necessary data and analytic outputs (see Figure 2).
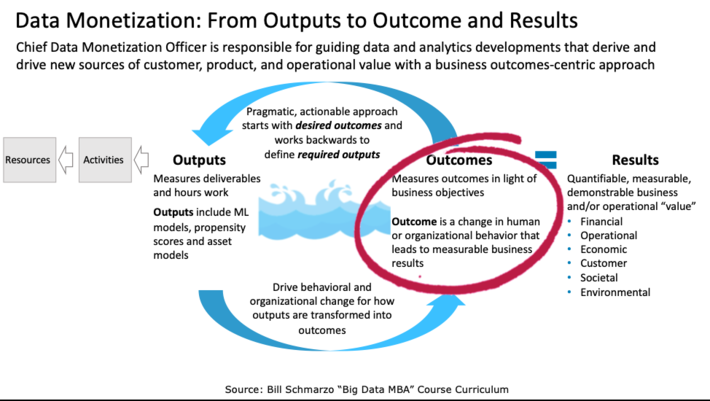
Figure 2: Defining the Data Monetization Role
The data monetization practice must transition the organization from just creating data and analytic outputs, to delivering business and operational outcomes that deliver meaningful and measurable business value.
Here are a few tools available to the CDMO to power the organization’s Data Monetization practice.
1) Data Lake 3.0: Collaborative Value Creation Platform
If you seek to exploit the unique characteristics of data – assets that never deplete, never wear out and can be used across an infinite number of use cases at zero marginal cost – then you must knock down the data silos that stymie the data economic multiplier effect. We can unleash the data economic multiplier effect by transforming the organization’s data lake into a “collaborative value creation” platform that supports the capture, sharing, re-use and continuous refinement of the organization’s data and analytic assets (see Figure 3).
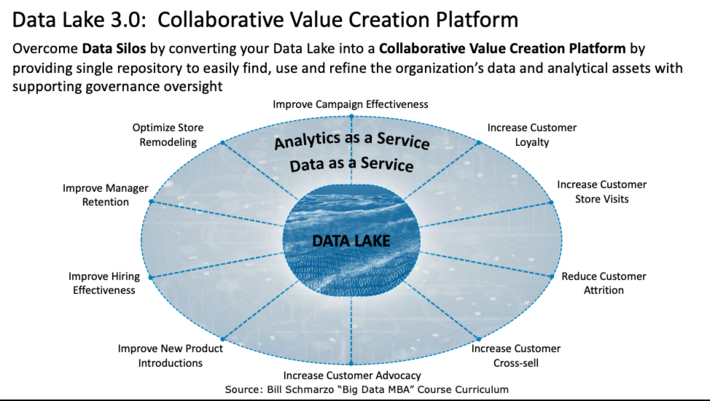
Figure 3: Data Lake 3.0: Collaborative Value Creation Platform
Note: The Data Economic Multiplier Effect drives the ability to share, re-use and refine the organization’s data assets across an unlimited number of use cases at near zero marginal cost.
2) Reusable, Continuously-Learning and Adapting Analytic Modules
I’ve written several times about the challenge of Orphaned Analytics, ML models that were built for a one-off business or operational need but never “engineered” for sharing, reuse and continuous refinement. Orphaned analytics are the great destroyers of the economic value of data and analytics because they hinder an organization’s ability to build analytic assets that appreciate, not depreciate, in value the more that they are used (see Figure 4).
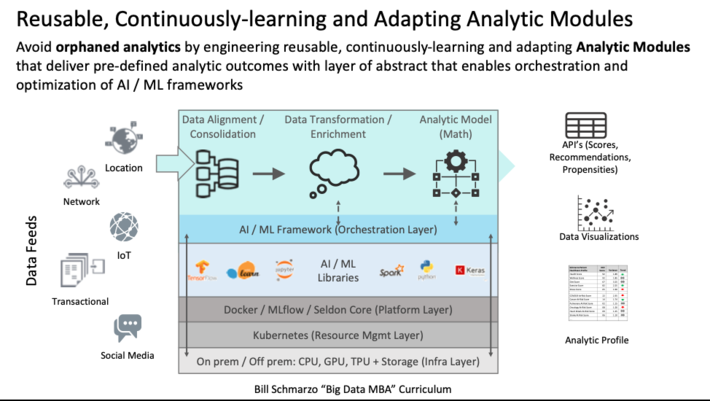
Figure 4: Engineering Reusable, Continuously-Learning and Adapting Analytic Modules.
Reusable, continuously-learning and adapting Analytic Modules can be created for the organization’s most common analytic needs (i.e., Anomaly Detection, Propensity to Act, Remaining Useful Life, Operator Effectiveness) and then can be linked together (using technologies such as Docker containers and Kubernetes) to address higher-value business and operational use cases such as reducing operational downtime, improving on-time delivery, reducing inventory, and improving customer retention.
3) Data Science Value Engineering Framework
If the goal of your organization’s data monetization governance efforts is to derive and drive new sources of customer, product and operational value, then those efforts must start by focusing on what’s important to the organization; that is, what are the organization’s most important business initiatives over the next 12 to 18 months, and how can data and analytics help to ensure success in those initiatives.
The Value Engineering Framework guides the business-data science collaboration to identify, validate, value and prioritize the key business and operational use cases that the organization needs to address in support of the targeted business initiative (see Figure 5).
Figure 5: Value Engineering Framework
Data Science Value Engineering process provides a simple yet effective methodology for exploiting the economic value of your data and analytics; to drive business and data science collaboration to apply data and analytics to improve operational and business effectiveness.
4) Hypothesis Development and Service Design Canvases
Design thinking provides valuable tools for driving cross-organizational collaboration and alignment in supporting the organization’s data monetization efforts. Two data science-based design templates in particular – Hypothesis Development Canvas and Service Design Canvas – are invaluable in ensuring that the organization’s data monetization efforts are focused on delivering meaningful business and operational outcomes (see Figure 6).
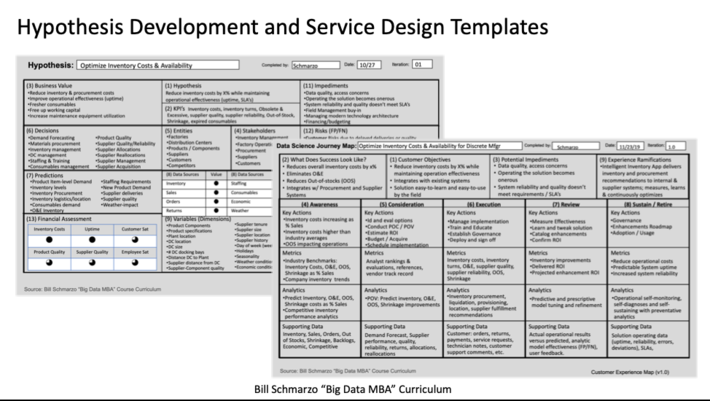
Figure 6: Data Science-based Design Templates
These design tools help identify, validate, value and prioritize the organization’s most important business and operational use cases, and the supporting data and analytic requirements.
Data (Governance) Monetization Practice Summary
The modern Data (Governance) Monetization practice must seek to drive cross-organizational co-creation to uncover, codify and operationalize new sources of customer, product, and operational value. This means mastering four fundamental concepts:
- Fundamental #1: Identify Sources of Value Creation. Leverages Design Thinking and Economics to identify, validate, value, and prioritize the sources of customer, product and operational value
- Fundamental #2: Codify Sources of Value Creation. Uses AI / ML to uncover and codify customer, product, and operational insights (propensities) into repeatable, continuously-learning and adapting Analytic Profiles and Analytic Modules
- Fundamental #3: Operationalize Sources of Value Creation. Creates continuous-learning feedback loop by embedding the customer, product and operational insights into the organization’s operational systems
- Fundamental #4: Data (Monetization) Governance. Creates data monetization governance discipline that optimizes the ML Model-to-Human collaboration that learns and adapts with every customer engagement or operational interaction
Your Data Monetization practice needs to spend less time thinking about data management and data governance, and more time thinking about data monetization governance. That’s the only way to ensure that your organization is sharing, re-using and continuously refining the unique economic characteristics of data and analytics to derive and drive new sources of customer, product, and operational value.
Final point, governance, to be successful, needs teeth. Governance must include rewards for compliance (e.g., resources, investments, budget, and executive attention) as well as penalties for non-compliance (e.g. withholding or even clawing back resources, investments, budget and executive attention). If your governance practice relies upon cajoling and begging others to comply, then your governance practice has already failed.
–
By Bill Schmarzo